Workshop #4
Hardware-Accelerated Efficient AI Applications
"Hardware/Software Optimization for Edge AI -- Two Sides of a Coin"
Dr. Giovanni ANSALONI (EPFL)
Abstract
This talk will focus on emerging opportunities and challenges brought by AI at the edge. I will argue that key to edge AI is hardware /software co-optimization. I will illustrate two complementary examples of this stance originating from a machine learning and a hardware design perspective, respectively. First, I will describe novel continual learning methodology tailored to cope with memory-constrained devices. Then, I will showcase how compute memories can disruptively increase performance and efficiency for AI workloads. Concluding my talk, I will introduce the X-HEEP environment, an extendible and open framework enabling the exploration and deployment of ultra-low-power AI systems and software.
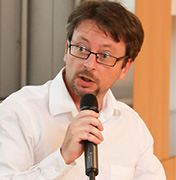
Dr. Giovanni ANSALONI (EPFL)
"Adapting Magnetoresistive Memory Devices for Unconventional Computing"
Prof. Qiming SHAO (HKUST)
Abstract
In the quest to evolve today's artificial intelligence systems into artificial general intelligence (AGI) that is capable of processing multimodality inputs, such as visual, vocal, and textual data. The limitations of conventional computing, like artificial neural networks, have become increasingly apparent. These methods rely on precise numerical computations from digital inputs and struggle with energy efficiency and deployment scalability. Exploring the unique properties of emerging devices to build multimodality unconventional computing architectures tailored for multimodal tasks in the AGI paradigm is critical. Magnetoresistive memory devices play an important role in this due to their stable and stochastic duality. The stable states of magnetic tunnel junctions provide reliable storage for computational parameters, while their stochastic switching behavior naturally supports probabilistic computing and the generation of probabilistic spikes. This dual functionality makes magnetoresistive memory devices ideally suited for enhancing AGI computing efficiency through unconventional methods.
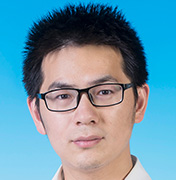
Prof. Qiming SHAO (HKUST)
"Early Timing and Power Evaluation for VLSI Design"
Prof. Zhiyao XIE (HKUST)
Abstract
As the integrated circuit (IC) complexity keeps increasing, the chip design cost is skyrocketing. Semiconductor companies are in increasingly greater demand for experienced manpower and are stressed with unprecedented turnaround time. There is a compelling need for design efficiency improvement through new electronic design automation (EDA) techniques. In this talk, I will present multiple design automation techniques based on machine learning (ML) methods, whose major strength is to explore highly complex correlations based on prior circuit data. These techniques cover the evaluation of primary chip-design objectives (i.e., performance and power) at very early design stages, including register-transfer level (RTL) and micro-architectural level. I will present challenges we observed in design quality evaluation at these early stages and our latest customized solutions.
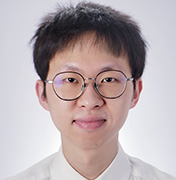
Prof. Zhiyao XIE (HKUST)