Public Lecture #1
"Can We Automate Accelerator Design with Deep Learning
Prof. Jason CONG (UCLA)
Abstract
Deep learning has shown promising results on many applications, such as image recognition, natural language processing, and protein folding. Recently, we started investigation of using deep learning to automate chip designs with a focus on design creation, leveraging our multi-decade research experience on high-level synthesis (HLS). In this talk, I present our latest progress on this topic. By coupling high-level synthesis with a set of deep learning techniques, such as graph-based neural networks, transfer learning, and large language models, we achieved promising results on both HLS quality prediction and design space exploration for general applications. When coupled microarchitecture guided optimization for regular structures, such as systolic arrays and stencil computation, we show that it is possible to automate IC designs so that most software programmers can design their own chips for a wide range of applications. This is an encouraging development, as there is a great need to design various kinds of customized hardware accelerators for better performance and energy efficiency, when we are approaching the end of Moore’s Law scaling.
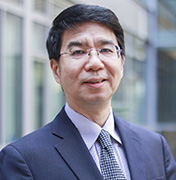
Prof. Jason CONG (UCLA)