Workshop #3 (RP-3)
Large Foundation AI Models for EDA and VLSI Design
"Large Language Model in EDA"
Prof. Bei YU (CUHK)
Abstract
In this talk, we explore the burgeoning intersection of Large Language Models (LLMs) and Electronic Design Automation (EDA). We critically assess whether LLMs represent a transformative future for EDA or merely a fleeting mirage. By analyzing current advancements, challenges, and potential applications, we dissect how LLMs can revolutionize EDA processes like design, verification, and optimization. Furthermore, we contemplate the ethical implications and feasibility of integrating these models into EDA workflows. Ultimately, this talk aims to provide a comprehensive, evidence-based perspective on the role of LLMs in shaping the future of EDA.
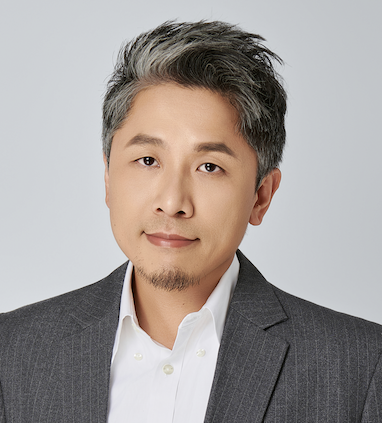
Prof. Bei YU (CUHK)
"AI-Assisted EDA: from Supervised Learning to Circuit Foundation Models"
Prof. Zhiyao XIE (HKUST)
Abstract
As the integrated circuit (IC) complexity keeps increasing, the chip design cost is skyrocketing. There is a compelling need for design efficiency improvement through new electronic design automation (EDA) techniques. As a result, AI-driven EDA techniques have been extensively explored for VLSI circuit design applications. In this talk, I will present the recent trend of developing foundation AI models for circuit design, also named circuit foundation models (CFMs). I will categorize existing circuit foundation models into two primary types: 1) encoder-based methods for general circuit representation learning for predictive tasks; and 2) decoder-based methods leveraging large language models (LLMs) for generative tasks. I will introduce representative works in each category, as well as our observed challenges and potential future research directions.
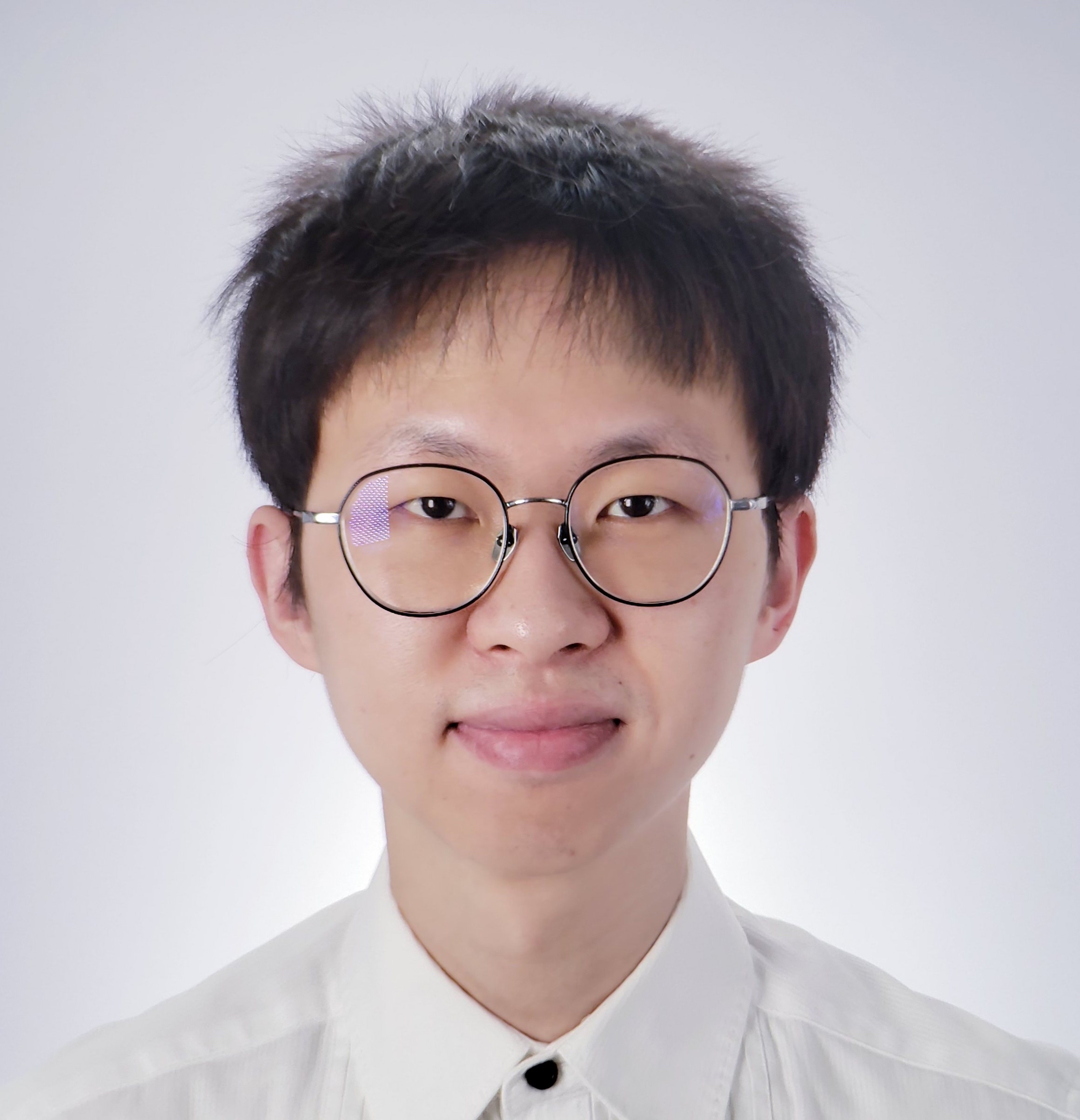
Prof. Zhiyao XIE (HKUST)
"Learning Correlated Topological and Geometrical Information from VLSI Circuit Layout Data"
Prof. Yibo LIN (PKU)
Abstract
Deep learning has been actively explored for early-stage design feedbacks and decision making in the VLSI circuit design flow. It can be applied to predict cross-stage design quality and guide optimization, especially for layout design stages like floorplan, placement, clock tree synthesis, routing, etc. As circuit layout involves correlated topological and geometrical information with extensive design optimization, it is a very unique problem and very challenging to learn the fine-grained correlation between such information across complicated design stages. In this talk, we present our recent efforts on learning correlated topological and geometrical information from VLSI circuit layout data using a large-scale AI-for-EDA dataset CircuitNet. We have explored various prediction tasks, like congestion, design rule violations, timing, and IR drop, demonstrating the potential of learning techniques in such scenarios.
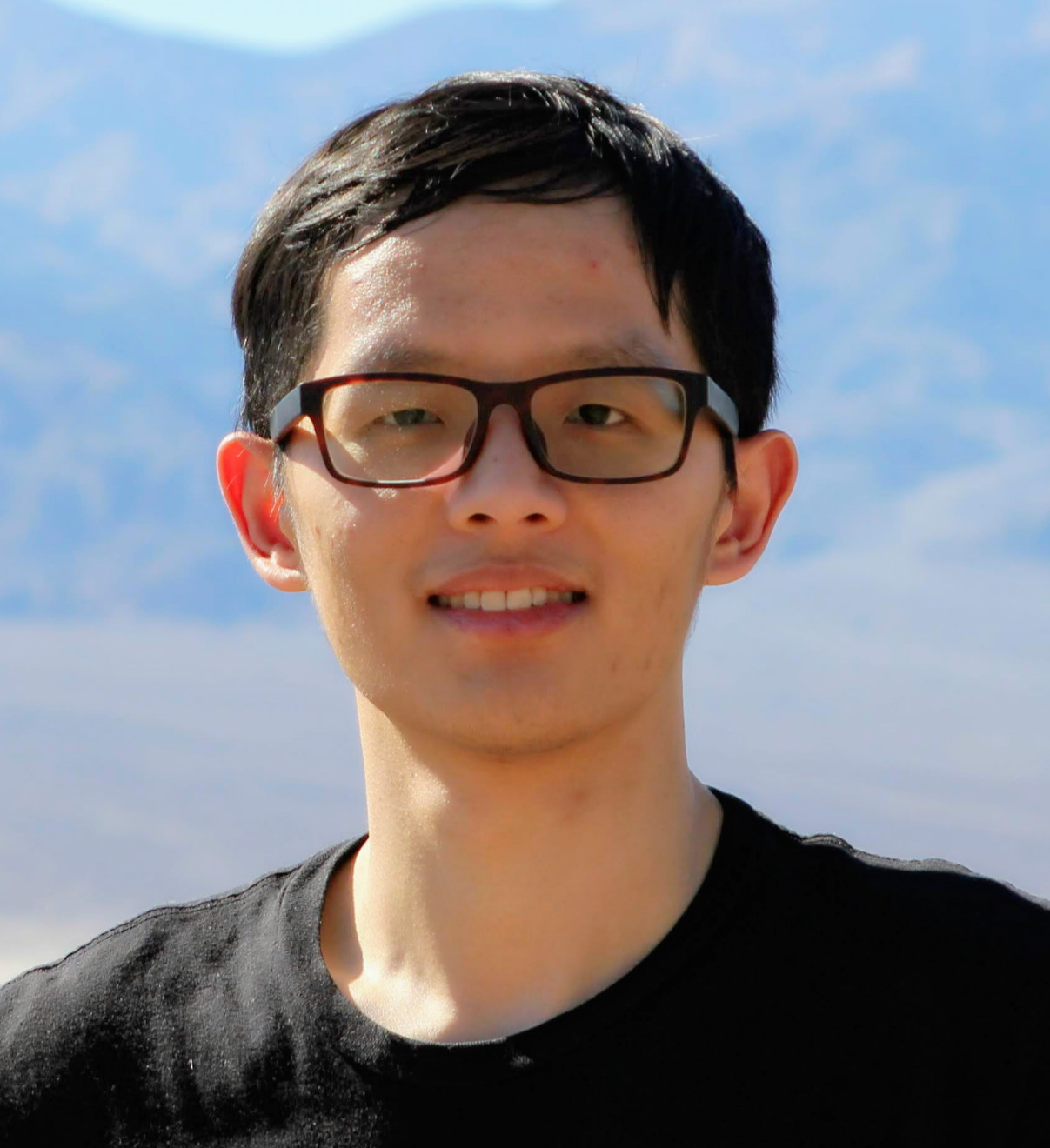
Prof. Yibo LIN (PKU)