Public Lecture #2
"Designing Accelerator-Centric Edge Architectures with Open-Source Platforms"
Prof. David ATIENZA ALONSO (École Polytechnique Fédérale de Lausanne (EPFL))
Abstract
Edge computing is targeting multiple domains nowadays, and a new set of complementary approaches have emerged as main avenues for designing novel accelerator-centric architectures for edge computing. The first approach is based on integrating accelerators in computing systems as part of the micro-architecture comprising validated open hardware components (processors, memories, and peripherals) to derive heterogeneous systems-on-chip. The second is to model the accelerator characteristics inside the edge architecture as a separate module that serves as co-processing system. In this talk, I will cover the pros and cons of these two approaches, focusing on recent works at the Embedded Systems Laboratory (ESL) of EPFL on the X-HEEP open hardware architectural template and the design of the ALPINE computing system for analog in-memory Acceleration with tight processor Integration. I will present these two systems, and describe how we employed these frameworks at ESL-EPFL with our industrial partners to explore emerging computation (e.g., analog vs. Digital in-memory computing) and communication (e.g., in-package wireless) paradigms to explore energy vs. performance vs. temperature control benefits in complex MPSoC architectures.
Reference
- Flavio Ponzina, Simone Machetti, Marco Rios, Benoît W. Denkinger, Alexandre. Levisse, Giovanni Ansaloni, Miguel Peon-Quiros, David Atienza, “A hardware/software co-design vision for deep learning at the edge”, IEEE Micro Magazine, ISSN: 0272-1732, Vol. 42, Issue no. 1, pp. 48 – 54, DOI: 10.1109/MM.2022.3195617, IEEE Press, December 2022. https://infoscience.epfl.ch/record/295432
- - Joshua Klein, Irem Boybat, Yasir Qureshi, Martino Dazzi, Alexandre Levisse, Giovanni Ansaloni, Marina Zapater, Abu Sebastian, and David Atienza, “ALPINE: Analog In-Memory Acceleration with Tight Processor Integration for Deep Learning”, IEEE Transactions on Computers (TC), 2023.: https://infoscience.epfl.ch/record/298771/
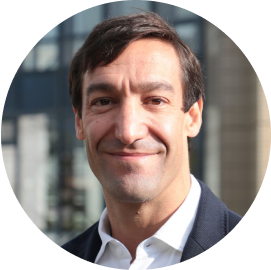
Prof. David ATIENZA ALONSO (École Polytechnique Fédérale de Lausanne (EPFL))